How Tech Can Help Predict Sports Results
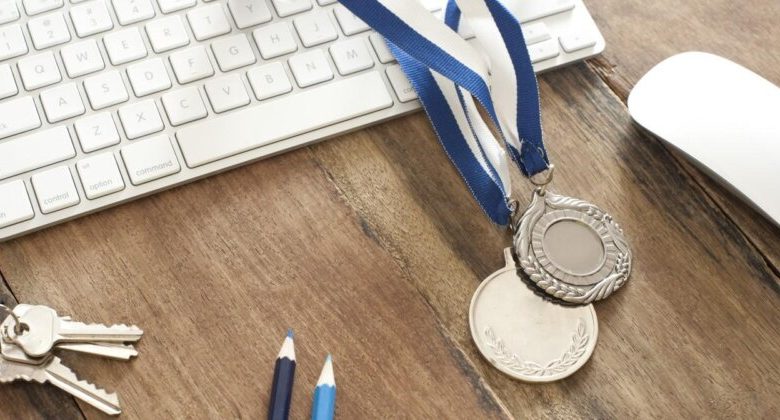

If you’re a follower of professional sports, you’ll likely already know all about the gambling industry’s recent impressive rise. Online betting has been popular in the United Kingdom and Ireland for many years. It has also proven popular in Canada, Australia, and several other prominent nations worldwide. Changes to the laws in the United States have brought sports gambling to a new audience, with millions of Americans now wagering on their favorite sports and competitions. Bookmakers cover the NFL, NBA, NHL, MLS, UFC, NASCAR, and other attractions.
Which is your favorite sport to watch on television? Do you enjoy the speed and power of the NFL, or perhaps you love the high-scoring nature of games from the NBA? Suppose you prefer soccer, like millions across the world. In that case, you’ll likely adore the English Premier League and the other top competitions from Europe, including the UEFA Champions League and the European Championships. Attend a game in person to see the stars in action or watch the play on television or the best live-streaming apps.
The top online bookmakers offer a long list of exciting markets and generous odds on soccer and other sports. You can wager on the match-winner, both teams to score, the handicap, the total goals or points, the first goalscorer, half-time/full-time, and others. Stick to one market or add a few favorites and create a Bet Builder. That option allows soccer lovers to add several markets to create a same-game parlay or accumulator bet.
How do you predict the result of a sports fixture? In the past, bettors spent many hours researching the stats and trends before finding the best odds and most generous promotions and confirming their predictions. Modern technology is changing how we research and settle on our picks. Keep reading as we explain how tech is helping sports fans call the winners more accurately and successfully than ever before.

Data
Data analytics is at the forefront of modern sports prediction. The sheer volume of data generated during sports events, from player statistics to environmental conditions, can be overwhelming. However, with the advent of big data technologies, this information can be harnessed effectively.
Detailed analysis of player performance metrics, such as speed, accuracy, and endurance, helps predict future performance. For instance, in football, data on passing accuracy, distance covered, and tackle success rates can indicate which team is more likely to win.
Analyzing historical data allows for the identification of trends and patterns. Teams or players with consistent past performance under similar conditions are more likely to repeat those performances.
Data on player injuries, recovery times, and their impact on performance can influence predictions. Knowing that a key player is out or not fully fit can significantly alter the expected outcome.
Tools
- SQL Databases: For storing and querying large datasets.
- R and Python: Popular programming languages for statistical analysis and predictive modeling.
- Tableau and Power BI: Visualization tools that help interpret complex data sets through interactive dashboards.
Machine learning
Machine learning (ML) and artificial intelligence (AI) have transformed the predictive analytics landscape by providing sophisticated algorithms that can learn from and make decisions based on data.
Algorithms like logistic regression, decision trees, and neural networks can be trained on historical data to predict outcomes. For instance, in basketball, models can predict game results by analyzing player stats, game conditions, and historical matchups.
AI can identify patterns that are not immediately obvious to human analysts. It includes recognizing tactical formations in football or shot patterns in tennis.
AI can process real-time data during events to update predictions dynamically. For example, changes in a cricket match’s win probability as the game progresses based on the current score and remaining overs.
Tools
- TensorFlow and PyTorch: Frameworks for building and training ML models.
- Scikit-learn: A Python library for simple and efficient tools for data mining and data analysis.
- IBM Watson: An AI platform that offers advanced data analytics capabilities.
Wearable Technology
Wearable technology and the Internet of Things (IoT) have introduced a new dimension to sports analytics by providing real-time data on athletes’ physical conditions.
Wearable devices track metrics such as heart rate, body temperature, and motion, providing insights into an athlete’s physical state and performance capacity.
Monitoring stress and fatigue levels helps in predicting potential injuries, allowing for preventive measures. This information can be critical for predicting a team’s performance if key players are at risk of injury.
Data collected from wearables can be used to optimize training regimes, enhance overall team performance, and thereby influence predictive models.
Tools
- Fitbit and Garmin: Wearable devices that track fitness and health metrics.
- Catapult Sports: Provides wearable tech specifically designed for athletes to track performance metrics.
- Smart Fabrics: Embedded sensors in clothing to monitor physiological data.
Social media
Social media platforms are treasure troves of information, and through sentiment analysis and trend identification, they can significantly influence sports predictions.
Algorithms can gauge public sentiment about teams, players, and even referees by analyzing social media posts, tweets, and fan forums. Positive or negative sentiment can impact a team’s morale and performance.
Tracking trending topics and hashtags can provide insights into injuries, team dynamics, and other factors that traditional data sources might miss.
The collective opinion of fans, especially those with deep knowledge of the sport, can be a powerful predictive tool. Platforms like Twitter often reveal insider information or subtle cues about team strategies and player conditions.
Tools
- Natural Language Processing (NLP): For analyzing and interpreting human language data.
- Social Media APIs: Tools like the Twitter API for extracting and analyzing data from social media platforms.
- Sentiment Analysis Tools: Software like Hootsuite Insights or Brandwatch for analyzing social media sentiment.
Simulation
Simulations and game theory provide advanced methodologies for predicting sports outcomes by modeling different scenarios and strategic interactions between teams.
These are used to model the probability of different outcomes based on random variables. For example, simulating thousands of iterations of a football match to predict likely scores.
This helps in understanding the strategic decisions made by teams and players. By modeling games as strategic interactions, analysts can predict the outcomes based on the participants’ optimal strategies.
Using simulations to pit teams against each other in a virtual environment to test various hypotheses and scenarios.
Tools
- MATLAB and Simulink: Platforms for performing simulations and mathematical modeling.
- Arena Simulation Software: For complex simulations in a variety of fields, including sports.
- AnyLogic: A multi-method simulation modeling tool.